The Rise of AIOps: Revolutionizing DevOps with Artificial Intelligence
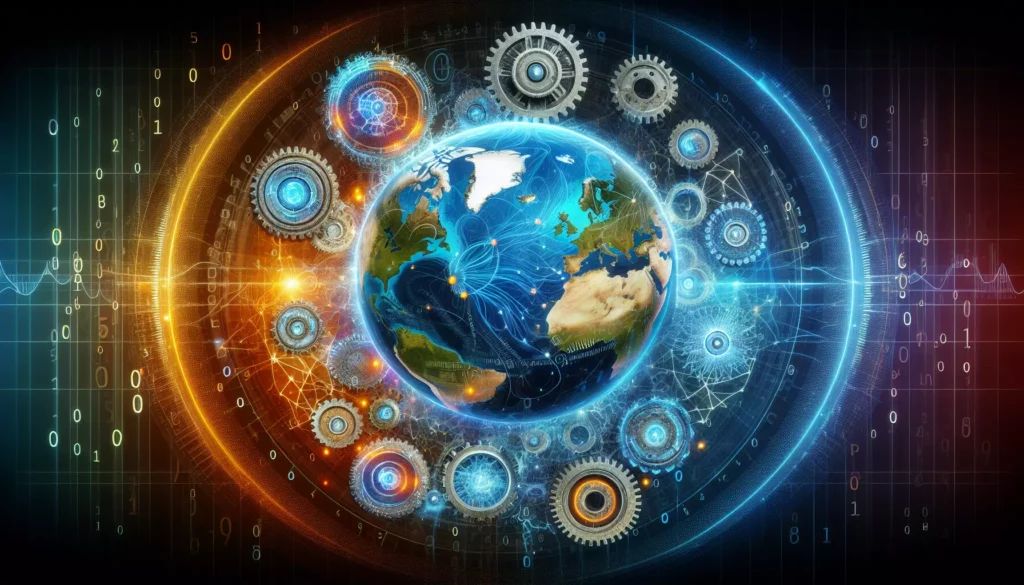
In the rapidly evolving world of software development and IT operations, the integration of Artificial Intelligence (AI) into DevOps practices has given rise to a powerful new paradigm known as AIOps (Artificial Intelligence for IT Operations). This innovative approach is transforming how organizations manage their IT infrastructure, automate processes, and enhance operational efficiency. In this comprehensive guide, we’ll explore the concept of AIOps, its benefits, implementation strategies, and its impact on the future of DevOps.
What is AIOps?
AIOps, short for Artificial Intelligence for IT Operations, refers to the application of machine learning, data analytics, and other AI technologies to automate and enhance IT operations. It combines big data and machine learning to automate IT operations processes, including event correlation, anomaly detection, and causality determination.
The term was coined by Gartner in 2017 and has since gained significant traction in the IT industry. AIOps platforms are designed to enhance and partially replace all primary IT operations functions, including availability and performance monitoring, event correlation and analysis, IT service management, and automation.
The Need for AIOps in Modern IT Environments
As IT infrastructures become increasingly complex and distributed, traditional DevOps practices are struggling to keep up with the volume, velocity, and variety of data generated by modern systems. This is where AIOps comes into play, addressing several key challenges:
- Data Overload: With the proliferation of microservices, containers, and cloud-native applications, the amount of data generated by IT systems has exploded. AIOps helps manage and make sense of this data deluge.
- Complexity: Modern IT environments are highly complex, with numerous interdependencies. AIOps can help identify patterns and relationships that humans might miss.
- Speed: The pace of change in IT operations is faster than ever. AIOps enables real-time analysis and rapid response to issues.
- Cost Efficiency: By automating routine tasks and improving decision-making, AIOps can significantly reduce operational costs.
- Proactive Problem Solving: AIOps can predict and prevent issues before they impact users, moving IT operations from a reactive to a proactive stance.
Key Components of AIOps
An effective AIOps platform typically consists of several key components:
1. Big Data
AIOps platforms ingest and process large volumes of data from various sources, including logs, metrics, and events. This data forms the foundation for analysis and decision-making.
2. Machine Learning
Machine learning algorithms are at the heart of AIOps. They analyze historical and real-time data to identify patterns, anomalies, and potential issues.
3. Automation
AIOps platforms automate routine tasks, incident response, and even complex workflows, reducing the need for manual intervention.
4. Visualization
Advanced visualization tools help present complex data and insights in an easily understandable format, enabling faster decision-making.
5. Integration
AIOps platforms integrate with existing IT tools and systems, providing a unified view of the entire IT environment.
Implementing AIOps: A Step-by-Step Guide
Implementing AIOps in your organization requires careful planning and execution. Here’s a step-by-step guide to help you get started:
Step 1: Assess Your Current IT Environment
Begin by evaluating your existing IT infrastructure, processes, and tools. Identify pain points and areas where AIOps could provide the most value.
Step 2: Define Clear Objectives
Establish specific goals for your AIOps implementation. These might include reducing mean time to resolution (MTTR), improving system uptime, or enhancing resource utilization.
Step 3: Choose the Right AIOps Platform
Select an AIOps platform that aligns with your objectives and integrates well with your existing tools. Consider factors such as scalability, ease of use, and vendor support.
Step 4: Start with a Pilot Project
Begin with a small-scale implementation to test the waters. This allows you to gain experience and demonstrate value before rolling out AIOps across the entire organization.
Step 5: Prepare Your Data
Ensure that your data is clean, properly formatted, and accessible. AIOps relies heavily on high-quality data for accurate insights.
Step 6: Train Your Team
Provide training to your IT staff on the new AIOps platform and processes. This is crucial for successful adoption and utilization of the technology.
Step 7: Implement and Iterate
Roll out your AIOps solution, monitor its performance, and continuously refine and optimize based on feedback and results.
AIOps in Action: Real-World Use Cases
To better understand the practical applications of AIOps, let’s explore some real-world use cases:
1. Anomaly Detection and Root Cause Analysis
AIOps platforms can quickly identify anomalies in system behavior and pinpoint the root cause of issues. For example, an e-commerce company used AIOps to detect a sudden spike in latency on their payment gateway. The system automatically correlated this with a recent software update, allowing the team to quickly rollback the change and restore normal operations.
2. Predictive Maintenance
By analyzing historical data and identifying patterns, AIOps can predict when equipment or systems are likely to fail. A telecommunications company implemented AIOps to predict network failures, allowing them to perform preventive maintenance and reduce downtime by 30%.
3. Automated Incident Response
AIOps can automate the response to common incidents, reducing the workload on IT teams. A large financial institution used AIOps to automate the resolution of routine password reset requests, freeing up their IT support team to focus on more complex issues.
4. Capacity Planning
AIOps can analyze usage patterns and predict future resource needs, enabling more effective capacity planning. A cloud service provider used AIOps to optimize resource allocation, resulting in a 25% reduction in infrastructure costs.
5. Security Threat Detection
AIOps can enhance cybersecurity by detecting and responding to threats in real-time. A government agency implemented AIOps to analyze network traffic patterns and identify potential security breaches, significantly improving their threat detection capabilities.
Challenges in Implementing AIOps
While AIOps offers numerous benefits, its implementation is not without challenges. Some common hurdles include:
1. Data Quality and Integration
AIOps relies heavily on high-quality, integrated data. Many organizations struggle with data silos and inconsistent data formats, which can hinder effective AIOps implementation.
2. Skill Gap
Implementing and managing AIOps requires specialized skills in areas such as machine learning and data science. Many organizations face a shortage of talent in these areas.
3. Cultural Resistance
Adopting AIOps often requires significant changes to existing processes and workflows. This can lead to resistance from team members who are comfortable with traditional methods.
4. Trust in AI-Driven Decisions
Building trust in AI-driven insights and automated actions can be challenging, especially in critical systems where the stakes are high.
5. Complexity of Implementation
Implementing AIOps can be complex, requiring careful planning, integration with existing systems, and ongoing maintenance and optimization.
The Future of AIOps
As AI and machine learning technologies continue to advance, the future of AIOps looks promising. Here are some trends and predictions for the evolution of AIOps:
1. Increased Automation
AIOps platforms will become more autonomous, handling an increasing number of tasks without human intervention. This will include not just incident response, but also proactive optimization of IT environments.
2. Enhanced Predictive Capabilities
As machine learning models become more sophisticated and have access to larger datasets, the predictive capabilities of AIOps will improve dramatically. This will enable more accurate forecasting of IT issues and resource needs.
3. Integration with Edge Computing
As edge computing becomes more prevalent, AIOps will evolve to manage and optimize distributed IT environments, including IoT devices and edge data centers.
4. Natural Language Processing (NLP) Integration
AIOps platforms will increasingly incorporate NLP capabilities, enabling more natural interactions between IT teams and AI systems. This could include chatbots for IT support and voice-controlled system management.
5. Explainable AI
As AI-driven decisions become more critical, there will be a greater focus on explainable AI in AIOps. This will help build trust and enable better collaboration between human operators and AI systems.
Best Practices for Successful AIOps Implementation
To maximize the benefits of AIOps and overcome potential challenges, consider the following best practices:
1. Start with Clear Objectives
Define specific, measurable goals for your AIOps implementation. This will help guide your efforts and measure success.
2. Ensure Data Quality
Invest in data cleansing and integration efforts to ensure your AIOps platform has access to high-quality, consistent data.
3. Foster a Culture of Continuous Learning
Encourage your team to embrace AI and machine learning technologies. Provide ongoing training and opportunities for skill development.
4. Start Small and Scale
Begin with a pilot project or specific use case, then gradually expand your AIOps implementation based on lessons learned.
5. Collaborate Across Teams
AIOps implementation should involve collaboration between IT operations, development teams, and business stakeholders to ensure alignment with organizational goals.
6. Continuously Monitor and Optimize
Regularly assess the performance of your AIOps implementation and make adjustments as needed to maximize its effectiveness.
Conclusion
AIOps represents a significant leap forward in the evolution of DevOps and IT operations. By harnessing the power of AI and machine learning, organizations can automate complex tasks, gain deeper insights into their IT environments, and proactively address issues before they impact users. While implementing AIOps comes with its challenges, the potential benefits in terms of efficiency, cost savings, and improved service quality make it a compelling proposition for modern IT organizations.
As we look to the future, AIOps is poised to play an increasingly central role in managing the complex, distributed IT environments that power our digital world. By embracing AIOps and following best practices for implementation, organizations can stay ahead of the curve and deliver more reliable, efficient, and innovative IT services.
Remember, the journey to AIOps is not just about technology—it’s about transforming how we approach IT operations and leveraging the power of AI to drive continuous improvement and innovation. As you embark on your AIOps journey, stay curious, remain adaptable, and always keep the end goal in sight: delivering exceptional value through optimized IT operations.