The Impact of Algorithms on Healthcare: Revolutionizing Patient Care and Medical Research
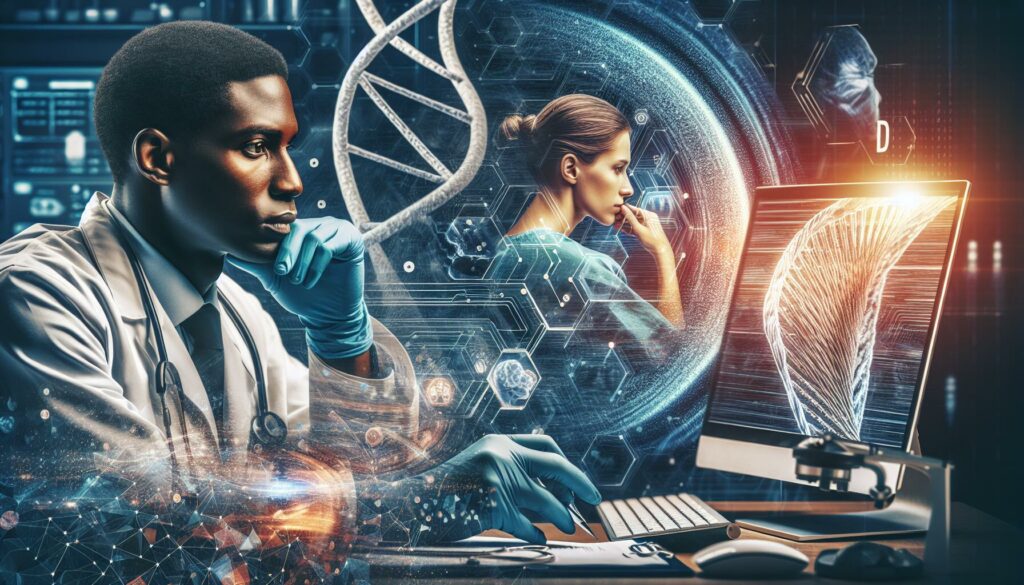
In recent years, the healthcare industry has undergone a significant transformation, largely due to the integration of advanced technologies and sophisticated algorithms. These algorithmic solutions are reshaping various aspects of healthcare, from diagnosis and treatment to drug discovery and patient care management. As we delve into this topic, we’ll explore how algorithms are revolutionizing healthcare and the profound impact they’re having on medical practices, patient outcomes, and the future of medicine.
Understanding Algorithms in Healthcare
Before we dive into the specifics, it’s essential to understand what we mean by algorithms in the context of healthcare. In simple terms, an algorithm is a step-by-step procedure for solving a problem or accomplishing a task. In healthcare, these algorithms are often complex mathematical models and computer programs designed to analyze vast amounts of medical data, recognize patterns, and make predictions or recommendations.
The algorithms used in healthcare can range from relatively simple decision trees to highly sophisticated machine learning and artificial intelligence systems. They are designed to process and interpret various types of medical data, including:
- Patient health records
- Medical imaging (X-rays, MRIs, CT scans)
- Genetic information
- Vital signs and real-time patient monitoring data
- Scientific literature and research findings
By leveraging these algorithms, healthcare professionals can gain valuable insights, make more informed decisions, and provide better care to their patients.
The Role of Algorithms in Diagnosis and Treatment
One of the most significant impacts of algorithms in healthcare is in the area of diagnosis and treatment. Advanced algorithmic systems are now capable of analyzing patient symptoms, medical history, and diagnostic test results to assist doctors in making more accurate diagnoses and developing effective treatment plans.
Improved Diagnostic Accuracy
Algorithms, particularly those powered by machine learning and artificial intelligence, have shown remarkable capabilities in improving diagnostic accuracy across various medical specialties. For example:
- Radiology: AI algorithms can analyze medical images with high precision, often detecting subtle abnormalities that human radiologists might miss. These algorithms can assist in identifying early signs of diseases like cancer, cardiovascular issues, and neurological disorders.
- Pathology: Machine learning algorithms can analyze tissue samples and help pathologists identify cancerous cells more accurately and efficiently.
- Dermatology: AI-powered image recognition algorithms can analyze skin lesions and help dermatologists differentiate between benign and malignant growths.
By augmenting human expertise with algorithmic analysis, healthcare providers can reduce the risk of misdiagnosis and ensure that patients receive appropriate treatment more quickly.
Personalized Treatment Plans
Algorithms are also playing a crucial role in developing personalized treatment plans. By analyzing a patient’s genetic information, medical history, lifestyle factors, and treatment responses, algorithmic systems can help doctors tailor treatments to individual patients. This approach, known as precision medicine, has the potential to significantly improve treatment outcomes and reduce adverse effects.
For instance, in oncology, algorithms can analyze a patient’s tumor genetics and recommend targeted therapies that are most likely to be effective for that specific cancer type. Similarly, in the field of psychiatry, algorithms can help predict which antidepressants are most likely to work for individual patients based on their genetic makeup and other factors.
Clinical Decision Support Systems
Algorithmic clinical decision support systems (CDSS) are becoming increasingly common in healthcare settings. These systems use algorithms to analyze patient data and provide recommendations to healthcare providers. They can:
- Alert doctors to potential drug interactions or allergies
- Suggest appropriate diagnostic tests based on patient symptoms
- Provide evidence-based treatment guidelines
- Identify high-risk patients who may need additional monitoring or interventions
By integrating these algorithmic support systems into their workflow, healthcare providers can make more informed decisions, reduce medical errors, and improve patient outcomes.
Algorithms in Medical Research and Drug Discovery
Beyond direct patient care, algorithms are also revolutionizing medical research and drug discovery processes. The ability of algorithms to analyze vast amounts of data and identify patterns is proving invaluable in advancing our understanding of diseases and developing new treatments.
Accelerating Drug Discovery
Traditional drug discovery is a time-consuming and expensive process, often taking years and billions of dollars to bring a new drug to market. Algorithms are helping to streamline this process in several ways:
- Virtual Screening: Machine learning algorithms can quickly screen millions of chemical compounds to identify those with the highest potential for treating specific diseases.
- Predictive Modeling: Algorithms can predict how drugs might interact with biological targets, helping researchers focus on the most promising candidates.
- Repurposing Existing Drugs: By analyzing the molecular structures and mechanisms of action of existing drugs, algorithms can identify potential new uses for these medications.
These algorithmic approaches are not only speeding up the drug discovery process but also making it more cost-effective, potentially leading to the development of new treatments for a wide range of diseases.
Analyzing Clinical Trial Data
Algorithms are also proving invaluable in the analysis of clinical trial data. They can:
- Identify patterns and trends that might not be apparent to human researchers
- Predict which patients are most likely to respond to a particular treatment
- Detect potential side effects or adverse reactions more quickly
- Help in the design of more efficient and effective clinical trials
By leveraging these algorithmic capabilities, researchers can conduct more targeted and efficient clinical trials, potentially bringing new treatments to patients more quickly.
Genomic Research
The field of genomics has been particularly transformed by the power of algorithms. Advanced machine learning algorithms can analyze vast amounts of genetic data to:
- Identify genetic markers associated with specific diseases
- Predict an individual’s risk of developing certain conditions
- Understand how genetic variations affect drug responses
- Explore the complex interactions between genes and environmental factors
These insights are paving the way for more personalized and effective healthcare strategies based on an individual’s genetic profile.
Enhancing Patient Care and Management
Beyond diagnosis and treatment, algorithms are also improving various aspects of patient care and healthcare management.
Predictive Analytics for Patient Risk
Algorithms can analyze patient data to predict which individuals are at high risk for certain conditions or complications. For example:
- Predicting which patients are likely to develop sepsis in hospital settings
- Identifying individuals at high risk of heart disease or stroke
- Forecasting which patients are most likely to be readmitted after discharge
By identifying high-risk patients, healthcare providers can implement preventive measures and interventions more effectively, potentially avoiding serious health issues and reducing healthcare costs.
Remote Patient Monitoring
With the rise of wearable devices and Internet of Things (IoT) technology, algorithms are playing a crucial role in remote patient monitoring. These systems can:
- Continuously analyze data from wearable devices to detect abnormal patterns
- Alert healthcare providers to potential issues before they become serious
- Help manage chronic conditions by tracking symptoms and medication adherence
- Provide personalized health recommendations based on real-time data
This algorithmic approach to patient monitoring is particularly valuable for managing chronic conditions and elderly care, allowing for more proactive and personalized healthcare interventions.
Healthcare Resource Optimization
On the administrative side, algorithms are helping healthcare organizations optimize their resources and improve operational efficiency. They can:
- Predict patient admission rates and help hospitals manage bed capacity
- Optimize staff scheduling based on predicted patient volumes
- Improve supply chain management for medical equipment and pharmaceuticals
- Enhance billing and claims processing accuracy
By improving operational efficiency, these algorithmic solutions can help healthcare providers reduce costs and improve the overall quality of care.
Ethical Considerations and Challenges
While the potential benefits of algorithms in healthcare are immense, their implementation also raises important ethical considerations and challenges that need to be addressed.
Data Privacy and Security
The use of algorithms in healthcare often requires access to large amounts of sensitive patient data. Ensuring the privacy and security of this data is paramount. Healthcare organizations must implement robust data protection measures and comply with regulations such as HIPAA in the United States or GDPR in Europe.
Moreover, there are concerns about the potential misuse of health data for purposes beyond patient care, such as insurance discrimination or targeted marketing. Clear guidelines and regulations are needed to protect patient privacy and ensure that health data is used ethically.
Algorithmic Bias
One of the significant challenges in implementing algorithms in healthcare is the potential for bias. If the data used to train these algorithms is not representative of diverse populations, the resulting models may perform poorly for certain groups or even exacerbate existing health disparities.
For example, if an algorithm is trained primarily on data from one ethnic group, it may not be as accurate in diagnosing or recommending treatments for patients from other ethnic backgrounds. Addressing this issue requires careful attention to data collection, algorithm design, and ongoing monitoring to ensure fairness and equity in healthcare delivery.
Transparency and Explainability
Many advanced algorithms, particularly those based on deep learning, operate as “black boxes,” making it difficult to understand how they arrive at their conclusions. This lack of transparency can be problematic in healthcare, where understanding the reasoning behind a diagnosis or treatment recommendation is crucial.
There is a growing emphasis on developing “explainable AI” in healthcare, which aims to make algorithmic decision-making processes more transparent and interpretable. This is essential for building trust among healthcare providers and patients, as well as for regulatory compliance.
Human-Algorithm Interaction
As algorithms become more prevalent in healthcare, there’s a need to carefully consider the balance between algorithmic recommendations and human judgment. While algorithms can process vast amounts of data and identify patterns that humans might miss, they lack the contextual understanding and empathy that human healthcare providers bring to patient care.
The challenge lies in developing systems that effectively augment human expertise rather than replace it, ensuring that the final decisions about patient care remain in the hands of qualified healthcare professionals.
The Future of Algorithms in Healthcare
Looking ahead, the role of algorithms in healthcare is likely to continue expanding and evolving. Some potential future developments include:
Advanced AI Diagnostics
As AI technologies continue to advance, we can expect to see even more sophisticated diagnostic tools that can analyze multiple data types simultaneously (e.g., combining imaging, genetic, and clinical data) to provide highly accurate and personalized diagnoses.
Predictive Health Modeling
Future algorithms may be able to create detailed, personalized health models for individuals, predicting their health trajectories and allowing for highly targeted preventive interventions.
AI-Assisted Surgery
While robots are already used in some surgical procedures, future algorithms may enable more autonomous surgical systems, capable of adapting to individual patient anatomy and making real-time decisions during operations.
Personalized Health Assistants
Advanced AI algorithms could power personalized health assistants that provide individuals with ongoing health advice, medication reminders, and early warning of potential health issues based on continuous monitoring of various health indicators.
Conclusion
The impact of algorithms on healthcare is profound and far-reaching. From improving diagnostic accuracy and personalizing treatments to accelerating medical research and enhancing patient care management, algorithms are transforming nearly every aspect of the healthcare industry.
However, as we continue to integrate these powerful tools into our healthcare systems, it’s crucial that we address the ethical considerations and challenges they present. Ensuring data privacy, mitigating algorithmic bias, improving transparency, and maintaining the right balance between human expertise and algorithmic insights will be key to realizing the full potential of algorithms in healthcare.
As we look to the future, it’s clear that algorithms will play an increasingly central role in healthcare. By embracing these technologies responsibly and thoughtfully, we have the opportunity to create a healthcare system that is more precise, efficient, and effective in improving patient outcomes and advancing medical knowledge.
The journey of integrating algorithms into healthcare is just beginning, and the potential benefits for patients, healthcare providers, and society as a whole are immense. As we continue to explore and refine these technologies, we move closer to a future where healthcare is not just reactive, but proactive, personalized, and powered by the incredible capabilities of algorithmic intelligence.