40 Powerful Techniques for Space Optimization in Algorithms
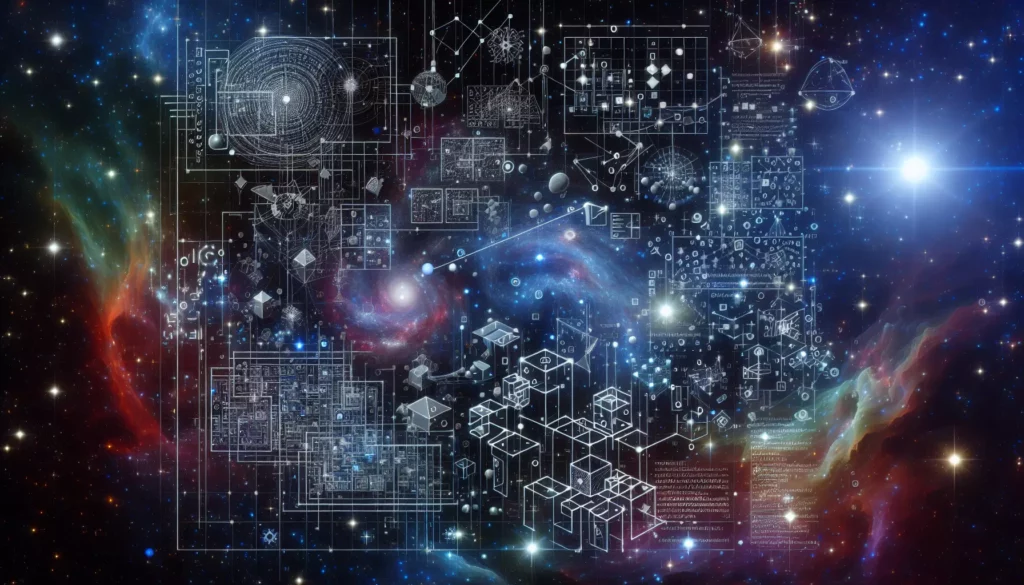
In the world of algorithm design and implementation, efficiency is key. While time complexity often takes center stage, space complexity is equally crucial, especially in resource-constrained environments. This comprehensive guide will explore 40 powerful techniques for space optimization in algorithms, helping you write more efficient and scalable code.
1. In-place Algorithms
In-place algorithms modify the input data structure directly without using additional space. This technique is particularly useful for sorting and array manipulation problems.
Example: In-place Reverse Array
def reverse_array(arr):
left, right = 0, len(arr) - 1
while left < right:
arr[left], arr[right] = arr[right], arr[left]
left += 1
right -= 1
return arr
# Usage
arr = [1, 2, 3, 4, 5]
reversed_arr = reverse_array(arr)
print(reversed_arr) # Output: [5, 4, 3, 2, 1]
2. Bit Manipulation
Using bit manipulation techniques can significantly reduce space usage, especially when dealing with boolean flags or small integer values.
Example: Checking if a number is even using bitwise AND
def is_even(num):
return (num & 1) == 0
# Usage
print(is_even(4)) # Output: True
print(is_even(7)) # Output: False
3. String Interning
String interning is a technique where only one copy of each distinct string is stored in memory. This can save space when dealing with many duplicate strings.
Example: String interning in Python
a = 'hello'
b = 'hello'
print(a is b) # Output: True (both variables reference the same string object)
4. Use of Generators
Generators allow you to iterate over a sequence of values without storing the entire sequence in memory.
Example: Using a generator for Fibonacci sequence
def fibonacci():
a, b = 0, 1
while True:
yield a
a, b = b, a + b
# Usage
fib = fibonacci()
for _ in range(10):
print(next(fib), end=' ')
# Output: 0 1 1 2 3 5 8 13 21 34
5. Memoization
Memoization involves caching the results of expensive function calls to avoid redundant computations. While it trades space for time, it can be more space-efficient than naive recursive solutions.
Example: Memoized Fibonacci
def memoized_fib(n, memo={}):
if n in memo:
return memo[n]
if n <= 1:
return n
memo[n] = memoized_fib(n-1, memo) + memoized_fib(n-2, memo)
return memo[n]
# Usage
print(memoized_fib(100)) # Calculates 100th Fibonacci number efficiently
6. Use of Constant Space
Some algorithms can be optimized to use only a constant amount of extra space, regardless of input size.
Example: Finding the majority element in an array
def majority_element(nums):
candidate = None
count = 0
for num in nums:
if count == 0:
candidate = num
count += (1 if num == candidate else -1)
return candidate
# Usage
arr = [2, 2, 1, 1, 1, 2, 2]
print(majority_element(arr)) # Output: 2
7. Space-Efficient Data Structures
Choosing the right data structure can significantly impact space usage. For example, using a Trie for storing strings with common prefixes can be more space-efficient than storing each string separately.
Example: Implementing a Trie
class TrieNode:
def __init__(self):
self.children = {}
self.is_end = False
class Trie:
def __init__(self):
self.root = TrieNode()
def insert(self, word):
node = self.root
for char in word:
if char not in node.children:
node.children[char] = TrieNode()
node = node.children[char]
node.is_end = True
def search(self, word):
node = self.root
for char in word:
if char not in node.children:
return False
node = node.children[char]
return node.is_end
# Usage
trie = Trie()
trie.insert("apple")
print(trie.search("apple")) # Output: True
print(trie.search("app")) # Output: False
8. Sliding Window Technique
The sliding window technique can be used to process subarrays or substrings efficiently without using extra space.
Example: Finding the maximum sum subarray of size k
def max_sum_subarray(arr, k):
n = len(arr)
if n < k:
return None
window_sum = sum(arr[:k])
max_sum = window_sum
for i in range(n - k):
window_sum = window_sum - arr[i] + arr[i + k]
max_sum = max(max_sum, window_sum)
return max_sum
# Usage
arr = [1, 4, 2, 10, 23, 3, 1, 0, 20]
k = 4
print(max_sum_subarray(arr, k)) # Output: 39
9. Two Pointer Technique
The two pointer technique can be used to solve various problems with minimal space usage, especially for array and linked list problems.
Example: Removing duplicates from a sorted array in-place
def remove_duplicates(nums):
if not nums:
return 0
i = 0
for j in range(1, len(nums)):
if nums[j] != nums[i]:
i += 1
nums[i] = nums[j]
return i + 1
# Usage
nums = [1, 1, 2, 2, 3, 4, 4, 5]
new_length = remove_duplicates(nums)
print(nums[:new_length]) # Output: [1, 2, 3, 4, 5]
10. Recursive Space Optimization
Tail recursion optimization can help reduce the space complexity of recursive algorithms by reusing stack frames.
Example: Tail-recursive factorial function
def factorial(n, acc=1):
if n == 0:
return acc
return factorial(n - 1, n * acc)
# Usage
print(factorial(5)) # Output: 120
11. Use of Static Variables
In some languages, using static variables can help reduce memory usage by sharing a single copy of the variable across all instances of a class.
Example: Using static variables in Java
public class Counter {
private static int count = 0;
public void increment() {
count++;
}
public int getCount() {
return count;
}
}
// Usage
Counter c1 = new Counter();
Counter c2 = new Counter();
c1.increment();
c2.increment();
System.out.println(c1.getCount()); // Output: 2
System.out.println(c2.getCount()); // Output: 2
12. Space-Time Tradeoffs
Sometimes, trading a little space for significant time improvements can be beneficial. For example, using a hash table for O(1) lookup time at the cost of O(n) space.
Example: Two Sum problem using a hash table
def two_sum(nums, target):
num_dict = {}
for i, num in enumerate(nums):
complement = target - num
if complement in num_dict:
return [num_dict[complement], i]
num_dict[num] = i
return []
# Usage
nums = [2, 7, 11, 15]
target = 9
print(two_sum(nums, target)) # Output: [0, 1]
13. Bitsets
Bitsets can be used to represent sets of small non-negative integers very space-efficiently.
Example: Implementing a simple bitset in Python
class Bitset:
def __init__(self, size):
self.bits = 0
self.size = size
def set(self, pos):
self.bits |= (1 << pos)
def clear(self, pos):
self.bits &= ~(1 << pos)
def test(self, pos):
return bool(self.bits & (1 << pos))
# Usage
bs = Bitset(8)
bs.set(1)
bs.set(4)
print(bs.test(1)) # Output: True
print(bs.test(2)) # Output: False
14. Sparse Matrix Representation
For matrices with many zero elements, using a sparse matrix representation can save significant space.
Example: Implementing a simple sparse matrix
class SparseMatrix:
def __init__(self, rows, cols):
self.rows = rows
self.cols = cols
self.elements = {}
def set(self, row, col, value):
if value != 0:
self.elements[(row, col)] = value
elif (row, col) in self.elements:
del self.elements[(row, col)]
def get(self, row, col):
return self.elements.get((row, col), 0)
# Usage
sm = SparseMatrix(1000, 1000)
sm.set(5, 10, 3.14)
print(sm.get(5, 10)) # Output: 3.14
print(sm.get(0, 0)) # Output: 0
15. Compression Techniques
Data compression can be used to reduce the space required to store or transmit data.
Example: Simple run-length encoding
def run_length_encode(s):
if not s:
return ""
result = []
count = 1
for i in range(1, len(s)):
if s[i] == s[i-1]:
count += 1
else:
result.append(f"{count}{s[i-1]}")
count = 1
result.append(f"{count}{s[-1]}")
return ''.join(result)
# Usage
print(run_length_encode("AABBBCCCC")) # Output: 2A3B4C
16. Memory Pooling
Memory pooling involves pre-allocating a large chunk of memory and managing it manually to avoid frequent allocations and deallocations.
Example: Simple object pool in Python
class ObjectPool:
def __init__(self, create_func, max_size):
self.create_func = create_func
self.max_size = max_size
self.pool = []
def acquire(self):
if self.pool:
return self.pool.pop()
return self.create_func()
def release(self, obj):
if len(self.pool) < self.max_size:
self.pool.append(obj)
# Usage
def create_expensive_object():
return [0] * 1000000 # Large list
pool = ObjectPool(create_expensive_object, 5)
obj1 = pool.acquire()
obj2 = pool.acquire()
pool.release(obj1)
obj3 = pool.acquire() # This will reuse obj1
17. Lazy Evaluation
Lazy evaluation defers the computation of values until they are actually needed, potentially saving space.
Example: Lazy evaluation using Python’s property decorator
class LazyComputation:
def __init__(self):
self._expensive_result = None
@property
def expensive_result(self):
if self._expensive_result is None:
print("Computing expensive result...")
self._expensive_result = sum(range(1000000))
return self._expensive_result
# Usage
lc = LazyComputation()
print(lc.expensive_result) # Computes and prints result
print(lc.expensive_result) # Uses cached result
18. Space-Efficient Sorting Algorithms
Some sorting algorithms, like Heapsort, can sort in-place with O(1) extra space.
Example: In-place Heapsort
def heapify(arr, n, i):
largest = i
left = 2 * i + 1
right = 2 * i + 2
if left < n and arr[largest] < arr[left]:
largest = left
if right < n and arr[largest] < arr[right]:
largest = right
if largest != i:
arr[i], arr[largest] = arr[largest], arr[i]
heapify(arr, n, largest)
def heapsort(arr):
n = len(arr)
for i in range(n // 2 - 1, -1, -1):
heapify(arr, n, i)
for i in range(n - 1, 0, -1):
arr[0], arr[i] = arr[i], arr[0]
heapify(arr, i, 0)
# Usage
arr = [12, 11, 13, 5, 6, 7]
heapsort(arr)
print(arr) # Output: [5, 6, 7, 11, 12, 13]
19. Circular Buffers
Circular buffers can be used to implement queues with fixed memory usage.
Example: Implementing a circular buffer
class CircularBuffer:
def __init__(self, size):
self.buffer = [None] * size
self.head = 0
self.tail = 0
self.size = size
self.count = 0
def enqueue(self, item):
if self.count == self.size:
raise Exception("Buffer is full")
self.buffer[self.tail] = item
self.tail = (self.tail + 1) % self.size
self.count += 1
def dequeue(self):
if self.count == 0:
raise Exception("Buffer is empty")
item = self.buffer[self.head]
self.head = (self.head + 1) % self.size
self.count -= 1
return item
# Usage
cb = CircularBuffer(3)
cb.enqueue(1)
cb.enqueue(2)
cb.enqueue(3)
print(cb.dequeue()) # Output: 1
cb.enqueue(4)
print(cb.dequeue()) # Output: 2
20. Bloom Filters
Bloom filters provide a space-efficient probabilistic data structure for set membership queries.
Example: Simple Bloom filter implementation
import mmh3
class BloomFilter:
def __init__(self, size, hash_count):
self.size = size
self.hash_count = hash_count
self.bit_array = [0] * size
def add(self, item):
for seed in range(self.hash_count):
result = mmh3.hash(item, seed) % self.size
self.bit_array[result] = 1
def check(self, item):
for seed in range(self.hash_count):
result = mmh3.hash(item, seed) % self.size
if self.bit_array[result] == 0:
return False
return True
# Usage
bf = BloomFilter(20, 3)
bf.add("apple")
bf.add("banana")
print(bf.check("apple")) # Output: True
print(bf.check("orange")) # Output: False (probably)
21. Prefix Sum Array
Prefix sum arrays can be used to efficiently compute range sums with O(1) space complexity after preprocessing.
Example: Range sum queries using prefix sum
def build_prefix_sum(arr):
prefix_sum = [0] * (len(arr) + 1)
for i in range(1, len(prefix_sum)):
prefix_sum[i] = prefix_sum[i-1] + arr[i-1]
return prefix_sum
def range_sum(prefix_sum, left, right):
return prefix_sum[right + 1] - prefix_sum[left]
# Usage
arr = [1, 2, 3, 4, 5]
prefix_sum = build_prefix_sum(arr)
print(range_sum(prefix_sum, 1, 3)) # Sum of arr[1:4], Output: 9
22. Segment Trees
Segment trees provide efficient range queries and updates with O(n) space complexity.
Example: Segment tree for range minimum queries
class SegmentTree:
def __init__(self, arr):
self.n = len(arr)
self.tree = [0] * (4 * self.n)
self.build(arr, 0, 0, self.n - 1)
def build(self, arr, node, start, end):
if start == end:
self.tree[node] = arr[start]
else:
mid = (start + end) // 2
self.build(arr, 2*node+1, start, mid)
self.build(arr, 2*node+2, mid+1, end)
self.tree[node] = min(self.tree[2*node+1], self.tree[2*node+2])
def query(self, node, start, end, l, r):
if r < start or end < l:
return float('inf')
if l <= start and end <= r:
return self.tree[node]
mid = (start + end) // 2
left = self.query(2*node+1, start, mid, l, r)
right = self.query(2*node+2, mid+1, end, l, r)
return min(left, right)
def range_minimum(self, l, r):
return self.query(0, 0, self.n-1, l, r)
# Usage
arr = [1, 3, 2, 7, 9, 11]
st = SegmentTree(arr)
print(st.range_minimum(1, 4)) # Output: 2
23. Fenwick Trees (Binary Indexed Trees)
Fenwick trees provide efficient range sum queries and updates with O(n) space complexity.
Example: Fenwick tree for range sum queries
class FenwickTree:
def __init__(self, n):
self.size = n
self.tree = [0] * (n + 1)
def update(self, i, delta):
while i <= self.size:
self.tree[i] += delta
i += i & (-i)
def sum(self, i):
s = 0
while i > 0:
s += self.tree[i]
i -= i & (-i)
return s
def range_sum(self, left, right):
return self.sum(right) - self.sum(left - 1)
# Usage
ft = FenwickTree(5)
arr = [1, 2, 3, 4, 5]
for i, val in enumerate(arr, 1):
ft.update(i, val)
print(ft.range_sum(2, 4)) # Output: 9
24. Suffix Arrays
Suffix arrays provide a space-efficient alternative to suffix trees for string processing tasks.
Example: Building a suffix array
def build_suffix_array(s):
suffixes = [(s[i:], i) for i in range(len(s))]
suffixes.sort()
return [i for _, i in suffixes]
# Usage
s = "banana"
sa = build_suffix_array(s)
print(sa) # Output: [5, 3, 1, 0, 4, 2]
25. Sparse Table
Sparse tables provide efficient range minimum/maximum queries with O(n log n) preprocessing and O(1) query time.
Example: Sparse table for range minimum queries
import math
def build_sparse_table(arr):
n = len(arr)
k = int(math.log2(n)) + 1
st = [[0] * k for _ in range(n)]
for i in range(n):
st[i][0] = arr[i]
for j in range(1, k):
for i in range(n - (1 << j) + 1):
st[i][j] = min(st[i][j-1], st[i + (1 << (j-1))][j-1])
return st
def query(st, L, R):
j = int(math.log2(R - L + 1))
return min(st[L][j], st[R - (1 << j) + 1][j])
# Usage
arr = [1, 3, 4, 8, 6, 1, 4, 2]
sparse_table = build_sparse_table(arr)
print(query(sparse_table, 2, 7)) # Output: 1
26. Union-Find (Disjoint Set)
Union-Find data structure provides efficient operations for maintaining disjoint sets with near-constant time complexity.
Example: Union-Find implementation
class UnionFind:
def __init__(self, n):
self.parent = list(range(n))
self.rank = [0] * n
def find(self, x):
if self.parent[x] != x:
self.parent[x] = self.find(self.parent[x])
return self.parent[x]
def union(self, x, y):
px, py = self.find(x), self.find(y)
if px == py:
return
if self.rank[px] < self.rank[py]:
self.parent[px] = py
elif self.rank[px] > self.rank[py]:
self.parent[py] = px
else:
self.parent[py] = px
self.rank[px] += 1
# Usage
uf = UnionFind(5)
uf.union(0, 1)
uf.union(2, 3)
uf.union(1, 2)
print(uf.find(0) == uf.find(3)) # Output: True
27. Space-Efficient Graph Representations
Choosing the right graph representation can significantly impact space usage.
Example: Adjacency list representation for sparse graphs
class Graph:
def __init__(self, vertices):
self.V = vertices
self.graph = [[] for _ in range(vertices)]
def add_edge(self, u, v):
self.graph[u].append(v)
self.graph[v].append(u) # For undirected graph
# Usage
g = Graph(4)
g.add_edge(0, 1)
g.add_edge(0, 2)
g.add_edge(1, 2)
g.add_edge(2, 3)
28. Succinct Data Structures
Succinct data structures aim to represent data using space close to the information-theoretic lower bound.
Example: Succinct representation of a binary tree
class SuccinctBinaryTree:
def __init__(self, tree_string):
self.tree = tree_string
def is_leaf(self, node):
return self.tree[node] == '1'
def left_child(self, node):
return 2 * node + 1
def right_child(self, node):
return 2 * node + 2
def parent(self, node):
return (node - 1) // 2
# Usage
tree = SuccinctBinaryTree("10110100")
print(tree.is_leaf(3)) # Output: True
29. Memory-Mapped Files
Memory-mapped files allow you to treat file content as if it were in memory, potentially saving RAM for large datasets.
Example: Using memory-mapped files in Python
import mmap
import os
def sum_integers_from_file(filename):
with open(filename, "r+b") as f:
mmapped_file = mmap.mmap(f.fileno(), 0)
total = sum(int(line) for line in mmapped_file)
mmapped_file.close()
return total
# Usage
# Assume "numbers.txt" contains one integer per line
print(sum_integers_from_file("numbers.txt"))
30. Streaming Algorithms
Streaming algorithms process data in a single pass, using sublinear space relative to the input size.
Example: Reservoir sampling for random sampling from a stream
import random
def reservoir_sampling(stream, k):
reservoir = []
for i, item in enumerate(stream):
if len(reservoir) < k:
reservoir.append(item)
else:
j = random.randint(0, i)
if j < k:
reservoir[j] = item
return reservoir
# Usage
stream = range(1000000)
sample = reservoir_sampling(stream, 10)
print(sample)
31. Lossy Counting
Lossy counting is a technique for approximating frequency counts in data streams with limited space.
Example: Lossy counting for frequent items
class LossyCounter:
def __init__(self, epsilon):
self.epsilon = epsilon
self.N = 0
self.counts = {}
self.bucket_id = 1
def add(self, item):
self.N += 1
if item in self.counts:
self.counts[item][0] += 1
elif len(self.counts) < 1 / self.epsilon:
self.counts[item] = [1, self.bucket_id - 1]
if self.N % int(1 / self.epsilon) == 0:
self.counts = {k: v for k, v in self.counts.items() if v[0] + v[1] > self.bucket_id}
self.bucket_id += 1
def get_frequent_items(self, support):
return {k: v[0] for k, v in self.counts.items() if v[0] > (support - self.epsilon) * self.N}
# Usage
lc = LossyCounter(0.1)
for item in [1, 2, 3, 1, 1, 2, 1, 1]:
lc.add(item)
print(lc.get_frequent_items(0.3)) # Output: {1: 5}
32. Probabilistic Data Structures
Probabilistic data structures trade perfect accuracy for space efficiency.
Example: HyperLogLog for cardinality estimation
import mmh3
import math
class HyperLogLog:
def __init__(self, p):
self.p = p
self.m = 1 << p
self.registers = [0] * self.m
self.alpha = self._get_alpha()
def _get_alpha(self):
if self.p == 4:
return 0.673
elif self.p == 5:
return 0.697
elif self.p == 6:
return 0.709
return 0.7213 / (1 + 1.079 / self.m)
def add(self, item):
x = mmh3.hash(item)
j = x & (self.m - 1)
w = x >> self.p
self.registers[j] = max(self.registers[j], self._get_rho(w))
def _get_rho(self, w):
return len(bin(w)) - 3
def count(self):
z = sum(math.pow